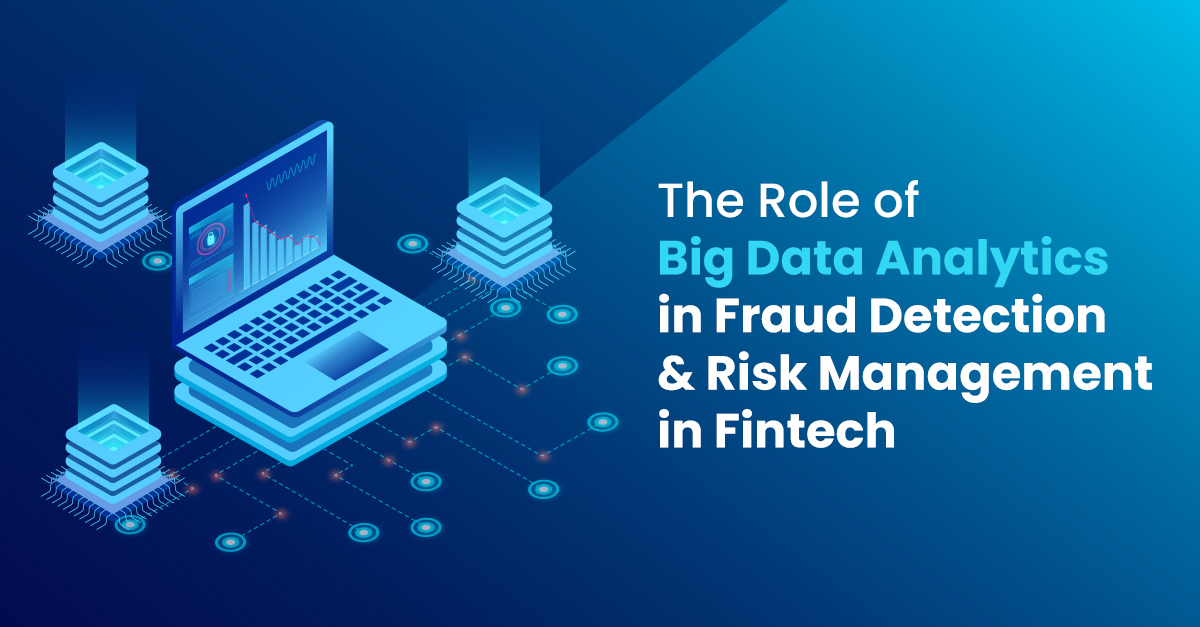
Table of Contents
Introduction
Combat fraud and manage risks in multiple settings…
Similar to as fraudsters are becoming more comprehensive in their attacks, so too are avenues companies can guard their data. Big data analytics is crucial for fraud detection and prevention as well as risk management. As per the Association of Certified Fraud Exmainers’ Reports to the Nations, organizations proactively using data monitoring can minimize their fraud losses by an average of about 54% and identify scams in half the time.
Big data analytics is alternating the patterns in which companies prevent fraud. AI, machine learning, and data mining tech stacks help counteract the hydra of fraud attempts affecting more than 3 billion identities each year.
In short, big data analytics techniques can help identify fraudulent activities and offer actionable reports used to monitor and prevent fraud- for businesses of all sizes.
What is Big Data Analytics?
Earlier companies used to rely upon manual reviews and employee tips to unfold fraud. Not so luckily, audits and informer reports often reveal fraud after the fact— that is, the scam was already successful. The manual process of hunting out fraud is also time-consuming, error-prone, and inefficient, missing a lot of instances concerning fraud that could have been otherwise preventable.
Big data analytics has completely revolutionised the entire process. It refers to the process of examining huge and complex data sets to uncover patterns, correlations, and other helpful information that can inform business decisions. The process uses specialized software and an algorithm to analyze huge amounts of data from multiple sources to identify signs of fraud and risks.
Big data analytics is a wide practice employed across multiple business functions. With the help of different types of data, companies can enhance their operations, minimize costs, and boost profits.
Why is Big Data Analytics Essential?
In this era where the amount of data is reaching a mesmerizing size, we continuously hear about big data analytics in every business and every system installed. So, why is important for companies?
Data analytics enables the analysis of huge volumes of data. Rather than driving decisions by analyzing large amounts of data over a good amount of time, a lot of quick decisions can be made with this data processed with a few techniques. Foresight may be required for the decisions to be made and in this instance, big data analytics makes it a lot easier to make predictions. Cheers to this, we can better understand customer requests.
For instance, the most visited sites, products, and most sold products are easily identifiable, and the data acquired from a survey on consumer opinions can be easily analyzed. Hence, proper products and services can be built and enhanced. Additionally, this processed and analyzed data can help develop algorithms that are likely to minimize the workload or time spent in the company. With the help of determined patterns, it guards the company from harm by identifying anomalies in the data. Though, big data analytics is now employed in almost every field, one of the most essential fields today is the banking and fintech sector giving rise to a focus on fraud analytics and risk analytics.
What Are Risk Analytics and Fraud Analytics?
Risk analytics employ data analytics to identify, assess, and manage risks. The process involves collecting and analyzing huge amounts of data to identify potential risks, evaluating the likelihood and impact of those risks, as well as creating a strategy to mitigate the highest-priority risks.
Fraud analytics, on the other hand, uses data analytics to identify and prevent fraud. It includes collecting and analyzing large amounts of data to recognize patterns and anomalies that might alarm credit card fraud, identify theft, insurance fraud, and other probable crimes.
A mind-boggling perk of big data analytics, and fraud and risk analytics, is that it enables the use of huge and complex data. Quick decisions can be made in real-time using data analytics techniques.
The Role of Big Data Analytics in Preventing and Detecting Frauds
Harnessing the power of big data needs vigorous infrastructure, advanced analytics techniques, and trained professionals to process, analyze, and obtain insights from large and diverse datasets.
Big data analytics is useful in preventing fraud using multiple techniques, like data mining, machine learning, and anomaly detection.
Here’s how big data can help prevent fraud:
- Recognizing patterns of fraudulent activity, like using stolen credit card numbers or making diverse petite payments in a short period.
- Anomaly detection in data, like if a customer all of a sudden initiates a large purchase that is out of character by their normal spending patterns, accessing their account from an unknown device.
- Mitigating fraud risk by recognizing and acknowledging the primary causes of fraud.
Big Data’s Influence Concerning Risk Management in Fintech
Big data has huge risk management potential as it delivers a more sophisticated and holistic view of potential dangers. It enables companies to identify trends, detect abnormalities, and unwrap hidden insights that traditional methods might miss.
Data Collection and Integration
Businesses need to have robust data collection and integration processes to exploit big data for risk management. They need to collect data from multiple sources and combine it in a common single database or data warehouse. Businesses can employ advanced data integration techniques to link data from different systems and sources, offering a unified perspective of hazards.
Predictive Modeling and Data Analysis
Predictive modelling and data analysis are vital components of big data risk management. Machine learning and predictive modelling are advanced analytics approaches that might find patterns, correlations, and trends in data. Businesses can develop prediction models that evaluate the chance of particular risks occurring by assessing historical data. This provides them with the proficiency to derive proactive preventive measures and develop risk mitigation plans.
Real-Time Risk Monitoring
Real-time risk monitoring is often facilitated by big data and analytics, enabling companies to respond quickly to dangers. Businesses can identify evolving dangers and drive quick action by continuously monitoring data sources and applying real-time analytics. Real-time monitoring enables proactive risk management and helps companies overlook or mitigate potential losses.
Conclusion
Technology is taking over the fintech companies at an unprecedented rate increasing the rate of risk prevailing in this domain. The application of Big data in the fintech industry, characterized by fraud detection, prevention, and risk management, has been successful in offering better solutions to the risks. It delivers a more accurate and precise prediction of their potential results.
Seize the frequency and severity of fraud and risk, so that you can identify and acknowledge issues before they become major issues.
With Smartinfologiks’s advanced, robust Analytics 101- the best business intelligence and analytics software, companies can run reports and identify trends. Smartinfologiks delivers a way to assess whether your fraud and risk management strategy is working.